Nearly every digital image that we see on the internet is edited in some way. From adjusting contrast to removing a stain on a shirt, software can help photographers bring images from ordinary to outstanding. But when editing crosses the line from sincere to sinister, it can shape viewers’ minds in dangerous ways.
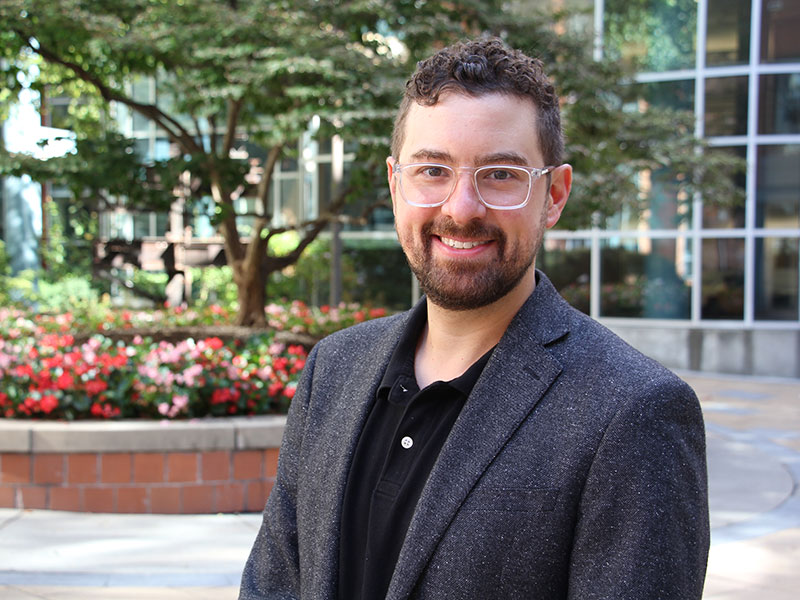
Matthew Stamm
Matthew Stamm, PhD, associate professor of electrical and computer engineering, is trying to shore up the defense against malicious editing. He is a specialist in information forensics, a growing field that seeks to determine when a piece of digital information is altered. And using machine learning, Stamm can teach computers to recognize identifying information about an image.
“The availability of powerful software that can create these fakes means that they can come from anywhere, and human investigators can’t keep up with the pace at which they are created,” Stamm says. “By teaching computers to recognize fakes, we may be able to stop many of them before they spread around the Internet.”
Stamm’s work has led to a partnership with the Defense Advanced Research Projects Agency. He was also research recently featured among Popular Science’s “Brilliant 10,” a list of the most innovative minds in science today. He was also featured in an online video about deepfakes produced by Vice.
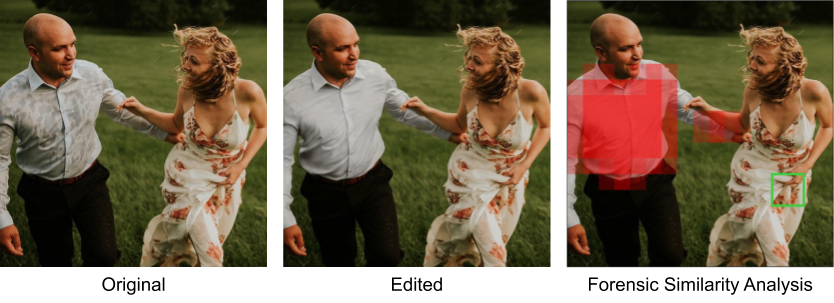
“Everything that touches a photo, from the camera that creates the image to the software you use to process it, leaves a digital fingerprint,” Stamm explains. “If contrast adjustment has been done to a photo, we can see the hallmarks of that in our analysis. We can even trace elements of the photo not just to the brand and model of camera that took it, but to the individual camera.”
“By teaching computers to recognize fakes, we may be able to stop many of them before they spread around the Internet.”
Matthew Stamm
Knowing this helps the algorithm see something else that’s increasingly important: By comparing a pixel’s identifying information to that of its neighbor, Stamm’s method can find parts of a photo that aren’t part of the original image. It can tell, for instance, when a face from one photo or video is placed into another from a completely different time and place to make it look like someone was somewhere they shouldn’t be. This is especially helpful in fighting “deepfakes” — digitally altered photos and videos that have been used to make it seem like politicians and other powerful people are saying something they didn’t.
As he continues his research, Stamm is already looking ahead to another important aspect of identifying deep fakes: teaching computers to make qualitative judgements about them.
“Once an image is flagged as fake, we need to know whether it’s malicious and whether it’s dangerous,” he says. “There’s a difference between putting one actor’s face onto another’s to imagine how they’d look in an iconic role and making it look like a world leader is declaring war. We need to be able to flag the latter kind of fake before it goes anywhere without spoiling the creativity of the people who make the former.”