On the Trail of Deepfakes, Drexel Researchers Identify ‘Fingerprints’ of AI-Generated Video

- U.S. Department of Education Provides Final Approval of Drexel and Salus Merger
- Spotting Bad Batteries Before They Malfunction
- When Climate Disasters Hit, They Often Leave Long-term Health Care Access Shortages, Drexel Study Finds
- New Research Highlights Health Benefits of Using Heritage Art Practices in Art Therapy
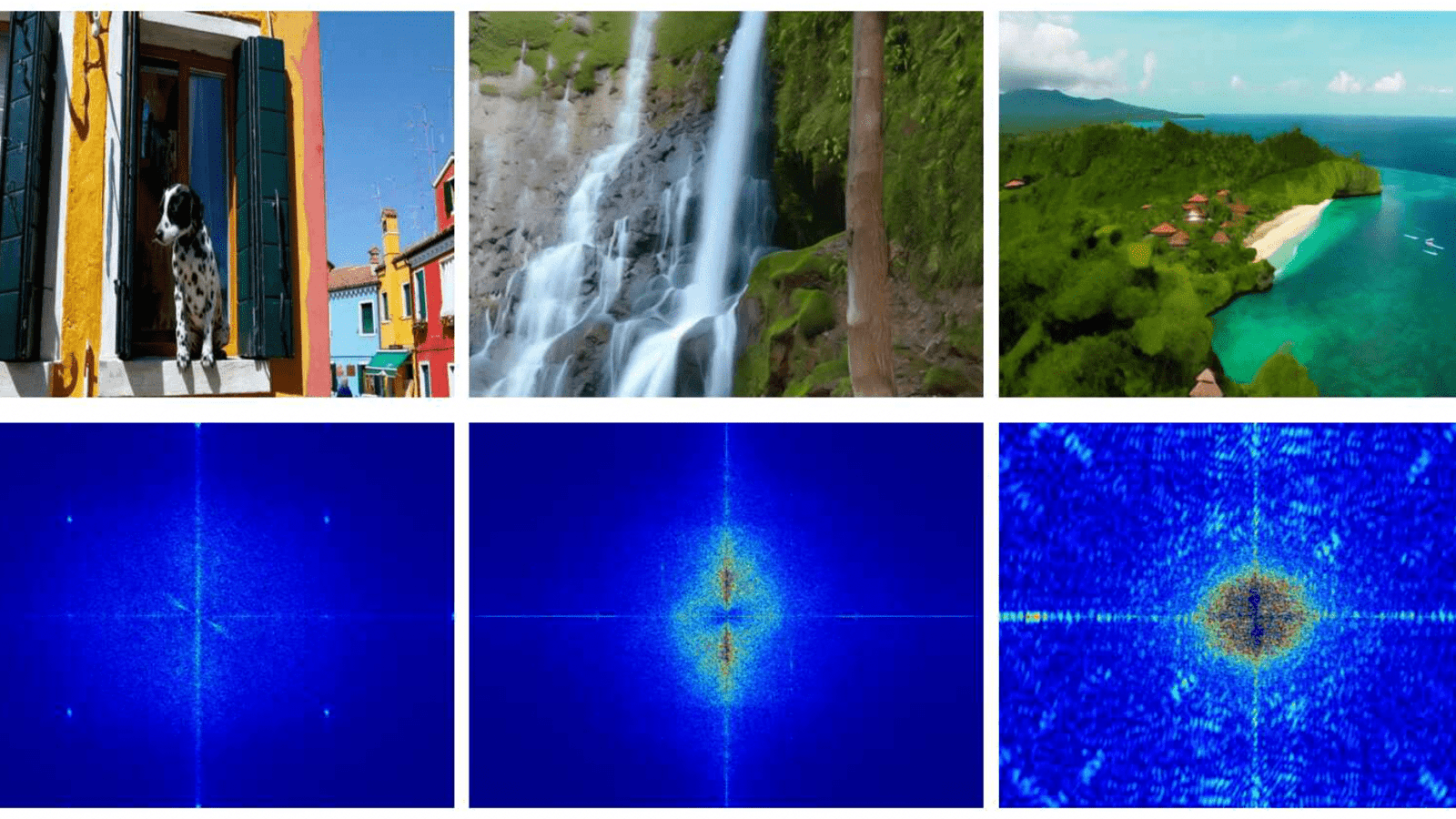
Research from Drexel University's College of Engineering suggests that current technology for detecting digitally manipulated images will not be effective in identifying new videos created by generative-AI technology. Frames from these videos (above) produce different forensic traces (below) than current detectors are calibrated to pick up.
In February, OpenAI released videos created by its generative artificial intelligence program Sora. The strikingly realistic content, produced via simple text prompts, is the latest breakthrough for companies demonstrating the capabilities of AI technology. It also raised concerns about generative AI’s potential to enable the creation of misleading and deceiving content on a massive scale. According to new research from Drexel University, current methods for detecting manipulated digital media will not be effective against AI-generated video; but a machine-learning approach could be the key to unmasking these synthetic creations.
In a paper accepted for presentation at the IEEE Computer Vision and Pattern Recognition Conference in June, researchers from Multimedia and Information Security Lab in Drexel’s College of Engineering explained that while existing synthetic image detection technology has failed thus far at spotting AI-generated video, they’ve had success with a machine learning algorithm that can be trained to extract and recognize digital “fingerprints” of many different video generators, such as Stable Video Diffusion, Video-Crafter and Cog-Video. Additionally, they have shown that this algorithm can learn to detect new AI generators after studying just a few examples of their videos.
“It’s more than a bit unnerving that this video technology could be released before there is a good system for detecting fakes created by bad actors,” said Matthew Stamm, PhD, an associate professor in Drexel’s College of Engineering and director of the MISL. “Responsible companies will do their best to embed identifiers and watermarks, but once the technology is publicly available, people who want to use it for deception will find a way. That’s why we’re working to stay ahead of them by developing the technology to identify synthetic videos from patterns and traits that are endemic to the media.”
Deepfake Detectives
Stamm’s lab has been active in efforts to flag digitally manipulated images and videos for more than a decade, but the group has been particularly busy in the last year, as editing technology is being used to spread political misinformation.
Until recently, these manipulations have been the product of photo and video editing programs that add, remove or shift pixels; or slow, speed up or clip out video frames. Each of these edits leaves a unique digital breadcrumb trail and Stamm’s lab has developed a suite of tools calibrated to find and follow them.
The lab’s tools use a sophisticated machine learning program called a constrained neural network. This algorithm can learn, in ways similar to the human brain, what is “normal” and what is “unusual” at the sub-pixel level of images and videos, rather than searching for specific predetermined identifiers of manipulation from the outset. This makes the program adept at both identifying deepfakes from known sources, as well as spotting those created by a previously unknown program.
The neural network is typically trained on hundreds or thousands of examples to get a very good feel for the difference between unedited media and something that has been manipulated — this can be anything from variation between adjacent pixels, to the order of spacing of frames in a video, to the size and compression of the files themselves.
A New Challenge
“When you make an image, the physical and algorithmic processing in your camera introduces relationships between various pixel values that are very different than the pixel values if you photoshop or AI-generate an image,” Stamm said. “But recently we’ve seen text-to video generators, like Sora, that can make some pretty impressive videos. And those pose a completely new challenge because they have not been produced by a camera or photoshopped.”
Last year a campaign ad circulating in support of Florida Gov. Ron DeSantis appeared to show former President Donald Trump embracing and kissing Antony Fauci was the first to use generative-AI technology. This means the video was not edited or spliced together from others, rather it was created whole-cloth by an AI program.
And if there is no editing, Stamm notes, then the standard clues do not exist — which poses a unique problem for detection.
“Until now, forensic detection programs have been effective against edited videos by simply treating them as a series of images and applying the same detection process,” Stamm said. “But with AI-generated video, there is no evidence of image manipulation frame-to-frame, so for a detection program to be effective it will need to be able to identify new traces left behind by the way generative-AI programs construct their videos.”
In the study, the team tested 11 publicly available synthetic image detectors. Each of these programs was highly effective — at least 90% accuracy — at identifying manipulated images. But their performance dropped by 20-30% when faced with discerning videos created by publicly available AI-generators, Luma, VideoCrafter-v1, CogVideo and Stable Diffusion Video.
“These results clearly show that synthetic image detectors experience substantial difficulty detecting synthetic videos,” they wrote. “This finding holds consistent across multiple different detector architectures, as well as when detectors are pretrained by others or retrained using our dataset.”
A Trusted Approach
The team speculated that convolutional neural network-based detectors, like its MISLnet algorithm, could be successful against synthetic video because the program is designed to constantly shift its learning as it encounters new examples. By doing this, it’s possible to recognize new forensic traces as they evolve. Over the last several years, the team has demonstrated MISLnet’s acuity at spotting images that had been manipulated using new editing programs, including AI tools — so testing it against synthetic video was a natural step.
“We’ve used CNN algorithms to detect manipulated images and video and audio deepfakes with reliable success,” said Tai D. Nguyen, a doctoral student in MISL, who was a coauthor of the paper. “Due to their ability to adapt with small amounts of new information we thought they could be an effective solution for identifying AI-generated synthetic videos as well.”
For the test, the group trained eight CNN detectors, including MISLnet, with the same test dataset used to train the image detectors, which including real videos and AI-generated videos produced by the four publicly available programs. Then they tested the program against a set of videos that included a number created by generative AI programs that are not yet publicly available: Sora, Pika and VideoCrafter-v2.
By analyzing a small portion — a patch — from a single frame from each video, the CNN detectors were able to learn what a synthetic video looks like at a granular level and apply that knowledge to the new set of videos. Each program was more than 93% effective at identify the synthetic videos, with MISLnet performing the best, at 98.3%.
The programs were slightly more effective when conducting an analysis of the entire video, by pulling out a random sampling of a few dozen patches from various frames of the video and using those as a mini training set to learn the characteristics of the new video. Using a set of 80 patches, the programs were between 95-98% accurate.
With a bit of additional training, the programs were also more than 90% accurate at identifying the program that was used to create the videos, which the team suggests is because of the unique, proprietary approach each program uses to produce a video.
“Videos are generated using a wide variety of strategies and generator architectures,” the researchers wrote. “Since each technique imparts significant traces, this makes it much easier for networks to accurately discriminate between each generator.”
A Quick Study
While the programs struggled when faced with the challenge of detecting a completely new generator without previously being exposed to at least a small amount of video from it, with a small amount of fine tuning MISLnet could quickly learn to make the identification at 98% accuracy. This strategy, called “few-shot learning” is an important capability because new AI technology is being created every day, so detection programs must be agile enough to adapt with minimal training.
“We’ve already seen AI-generated video being used to create misinformation,” Stamm said. “As these programs become more ubiquitous and easier to use, we can reasonably expect to be inundated with synthetic videos. While detection programs shouldn’t be the only line of defense against misinformation — information literacy efforts are key — having the technological ability to verify the authenticity of digital media is certainly an important step.”
This research was funded by DARPA, Air Force Research Laboratory (AFRL), and the National Science Foundation (NSF) In addition to Stamm and Nguyen, Danial Samadi Vahdati and Aref Azizpour, doctoral students in the College of Engineering, contributed to this paper.
Read the full paper here: https://ductai199x.github.io/beyond-deepfake-images/
Drexel News is produced by
University Marketing and Communications.