The Future of Artificial Intelligence Requires the Guidance of Sociology
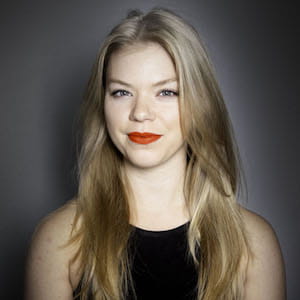
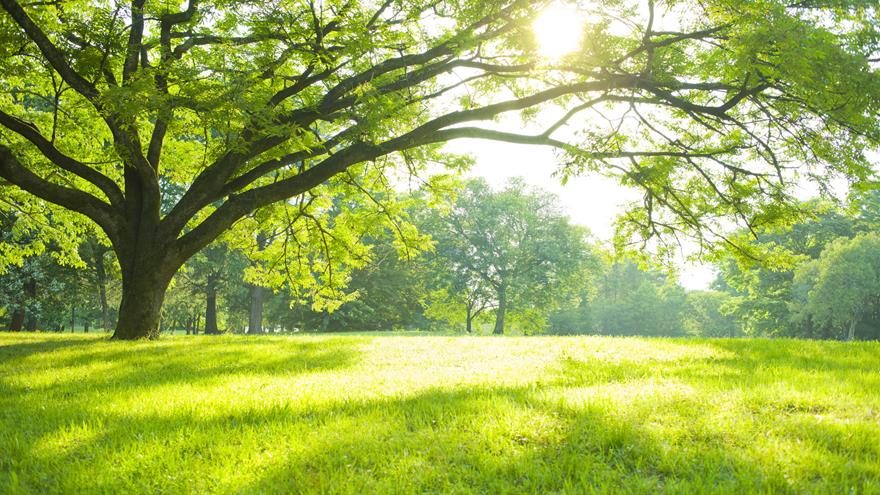
- Men and Residents of Higher Crime Areas See Greater Benefit from Community Parks, in Reduction of Deaths from Heart Disease
- Happy New Year 2025! Try These Proven Lifestyle Tips to Make Your New Year Happier.
- Faculty Highlights: Recent Awards and Grants
- A Framework for Community-Driven Environmental Justice Guided by the Community
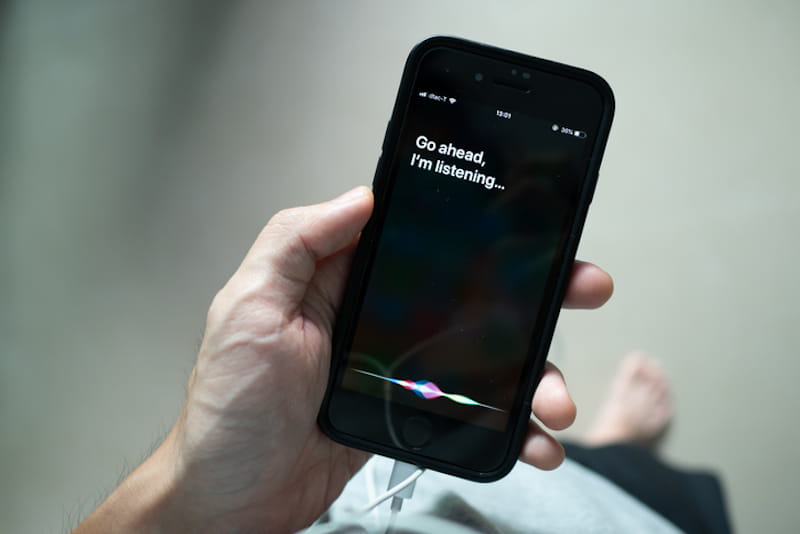
In the race to out-compete other companies– artificial intelligence (AI) design is lacking a deep understanding of what data about humans mean and its relation to equity. Two Drexel University sociologists suggest we pay greater attention to the societal impact of AI, as it is appearing more frequently than ever before.
“The coronavirus pandemic has sped up the use of AI and automation to replace human workers, as part of the effort to minimize the risks associated with face-to-face interactions,” said Kelly Joyce, PhD, a professor in the College of Arts and Sciences and founding director of the Center for Science, Technology and Society at Drexel. “Increasingly we are seeing examples of algorithms that are intensifying existing inequalities. As institutions such as education, healthcare, warfare, and work adopt these systems, we must remediate this inequity.”
In a newly published paper in Socius, Joyce, Susan Bell, PhD, a professor in the College of Arts and Sciences,and colleagues raise concerns about the push to rapidly accelerate AI development in the United States without accelerating the training and development practices necessary to make ethical technology. The paper proposes a research agenda for a sociology of AI.
“Sociology's understanding of the relationship between human data and long-standing inequalities is needed to make AI systems that promote equality,” explained Joyce.
How do we define AI?
The term AI has been used in many different ways and early interpretations associate the term with software that is able to learn and act on its own. For example, self-driving cars learn and identify routes and obstacles – just as robotic vacuums do the perimeter or layout of a home, and smart assistants (Alexa or Google Assistant) identify the tone of voice and preferences of their user.
“AI has a fluid definitional scope that helps explain its appeal,” said Joyce. “Its expansive, yet unspecified meaning enables promoters to make future-oriented, empirically unsubstantiated, promissory claims of its potential positive societal impact.”
Joyce, Bell and colleagues explain that in recent years, programming communities have largely focused on developing machine learning (ML) as a form of AI. The term ML is more commonly used among researchers than the term AI, although AI continues to be the public-facing term used by companies, institutes, and initiatives. “ML emphasizes the training of computer systems to recognize, sort, and predict outcomes from analysis of existing data sets,” explained Joyce.
AI practitioners, computer scientists, data scientists and engineers are training systems to recognize, sort and predict outcomes from analysis of existing data sets. Humans input existing data to help train AI systems to make autonomous decisions. The problem here is that AI practitioners do not typically understand how data about humans is almost always also data about inequality.
“AI practitioners may not be aware that data about X (e.g., ZIP codes, health records, location of highways) may also be data about Y (e.g., class, gender or race inequalities, socioeconomic status),” said Joyce, who is the lead author on the paper. “They may think, for example, that ZIP codes are a neutral piece of data that apply to all people in an equal manner instead of understanding that ZIP codes often also provide information about race and class due to segregation. This lack of understanding has resulted in the acceleration and intensification of inequalities as ML systems are developed and deployed."
“Identifying correlations between vulnerable groups and life chances, AI systems accept these correlations as causation, and use them to make decisions about interventions going forward. In this way, AI systems do not create new futures, but rather replicate the durable inequalities that exist in a particular social world,” explains Joyce.
Is AI imperiled by systemic racism and human bias?
There are politics tied to algorithms, data and code. Consider the search engine Google. Although Google search results might appear to be neutral or singular outputs, Google’s search engine recreates the sexism and racism found in everyday life.
“Search results reflect the decisions that go into making the algorithms and codes, and these reflect the standpoint of Google workers,” explains Bell. “Specifically, their decisions about what to label as sexist or racist reflect the broader social structures of pervasive racism and sexism. In turn, decisions about what to label as sexist or racist ‘trains’ an ML system. Although Google blames users for contributing to sexist and racist search results, the source lies in the ‘input.’”
Bell points out “in contrast to the perceived neutrality of Google’s search results, societal oppression and inequality are embedded in and amplified by them.”
Another example the authors point out are AI systems that use data from patients' electronic health records (EHRs) to make predictions about appropriate treatment recommendations. Although computer scientists and engineers often consider privacy when designing AI systems, understanding the multivalent dimensions of human data is not typically part of their training. Given this, they may assume that EHR data represents objective knowledge about treatment and outcomes, instead of viewing it through a sociological lens that recognizes how EHR data is partial and situated.
"When using a sociological approach," Joyce explains, "You understand that patient outcomes are not neutral or objective — these are related to patients’ socioeconomic status, and often tell us more about class differences, racism and other kinds of inequalities than the effectiveness of particular treatments."
The paper notes examples such as an algorithm that recommended that black patients receive less health care than white patients with the same conditions and a report showing that facial recognition software is less likely to recognize people of color and women showed that AI can intensify existing inequalities.
“A sociological understanding of data is important, given that an uncritical use of human data in AI sociotechnical systems will tend to reproduce, and perhaps even exacerbate, preexisting social inequalities,” said Bell. “Although companies that produce AI systems hide behind the claim that algorithms or platform users create racist, sexist outcomes, sociological scholarship illustrates how human decision making occurs at every step of the coding process.”
In the paper, the researchers demonstrate that sociological scholarship can be joined with other critical social science research to avoid some of the pitfalls of AI applications. “By examining the design and implementation of AI sociotechnical systems, sociological work brings human labor and social contexts into view,” said Joyce. Building on sociology’s recognition of the importance of organizational contexts in shaping outcomes, the paper shows that both funding sources and institutional contexts are key drivers of how AI systems are developed and used.
Does AI require the guidance of sociology? Researchers say, yes.
Joyce, Bell and colleagues suggest that, despite well-intentioned efforts to incorporate knowledge about social worlds into sociotechnical systems, AI scientists continue to demonstrate a limited understanding of the social — prioritizing that which may be instrumental for the execution of AI engineering tasks, but erasing the complexity and embeddedness of social inequalities.
“Sociology’s deeply structural approach also stands in contrast to approaches that highlight individual choice,” said Joyce. “One of the most pervasive tropes of political liberalism is that social change is driven by individual choice. As individuals, the logic goes, we can create more equitable futures by making and choosing better products, practices, and political representatives. The tech world tends to sustain a similarly individualistic perspective when its engineers and ethicists emphasize eliminating individual-level human bias and improving sensitivity training as a way to address inequality in AI systems.”
Joyce, Bell and colleagues invite sociologists to use the discipline’s theoretical and methodological tools to analyze when and how inequalities are made more durable by AI systems. The researchers emphasize that the creation of AI sociotechnical systems is not simply a question of technological design, but also raises fundamental questions about power and social order.
“Sociologists are trained to identify how inequalities are embedded in all aspects of society and to point toward avenues for structural social change. Therefore, sociologists should play a leading role in the imagining and shaping of AI futures,” said Joyce.
Drexel News is produced by
University Marketing and Communications.